Dr. Jonas Rieger
Contact
TU Dortmund University
Department of Statistics
Chair of Business and Social Statistics
CDI Building, Room 3
44221 Dortmund
Germany
E-Mail: riegerstatistik.tu-dortmundde
Phone: +49 231 755 5216
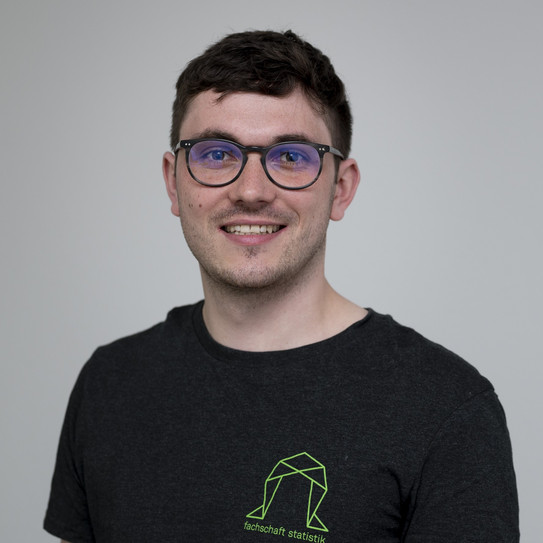
- since October 2022: Postdoc
- 2022-2023: NLP Scientist at the Leibniz Institute for Media Research | Hans-Bredow-Institut (HBI)
- 2018-2022: Doctorate in Statistics, TU Dortmund University
- 2016-2018: Research Assistant in the field of Text Mining
- 2016-2018: M.Sc. Statistics with minor in Computer Science, TU Dortmund University
- 2013-2016: B.Sc. Statistics with minor in Computer Science, TU Dortmund University
Projects:
- Project leader of Social (in)justice in the energy transition - from the digital debate to the living world; Subproject: Monitoring narratives about the energy transition.
- Engaged in the MERCUR project NEAR: Narrative Economics Alliance Ruhr.
- Funding from the TU Dortmund Young Academy for the project The Era of ChatGPT: Evaluation and Regulation of Large Language Models.
- Contributing to the EU project GADMO: German-Austrian Digital Media Observatory.
- Contributing to the BMBF project FLACA: Few-Shot Learning for Automated Content Analysis in Communication Science.
- Member of the Dortmund Center for data-based Media Analysis (DoCMA).
Interests:
- Topic models
- Evaluation (quality and reliability)
- Model selection and parameter tuning
- Update algorithms
- Detection of structural breaks, events and narratives in text corpora
- Few-shot text classification
- Adapters for transformers in few-shot scenarios
- Argument mining in news and social media discourses
- Text corpus-based indicators
- Characteristics of disinformation and fake news dissemination
- Content analysis of political texts and tweets
- topiclabels ([cran][github])
- rollinglda ([cran][github])
- ldaPrototype ([cran][github][joss])
- tosca ([cran][github])
- spINAR ([cran][github][joss])
Please also have a look at the PsychTopics App, which is based on the RollingLDA method as well as The World of Topic Modeling in R (Wiedemann, 2022) for a short overview of several R packages dealing with topic modeling.
- Rieger, J., Jentsch, C. and Rahnenführer, J. (2024). LDAPrototype: A model selection algorithm to improve reliability of latent Dirichlet allocation. PeerJ Computer Science 10(2279). DOI.
- Lange, K.-R., Rieger, J. and Jentsch, C. (2024). Lex2Sent: A bagging approach to unsupervised sentiment analysis. Proceedings of the 20th KONVENS Conference, 281-291. Link.
- Faymonville, M., Riffo, J., Rieger, J. and Jentsch, C. (2024). spINAR: An R Package for Semiparametric and Parametric Estimation and Bootstrapping of Integer-Valued Autoregressive (INAR) Models. Journal of Open Source Software 9(97), 5386. DOI.
- Rieger, J., Yanchenko, K., Ruckdeschel, M., von Nordheim, G., Kleinen-von Königslöw, K. and Wiedemann, G. (2024). Few-shot learning for automated content analysis: Efficient coding of arguments and claims in the debate on arms deliveries to Ukraine. Studies in Communication and Media 13, 72-100. DOI.
- Krause, C., Rieger, J., Flossdorf, J., Jentsch, C. and Beck, F. (2023). Visually Analyzing Topic Change Points in Temporal Text Collections. Vision, Modeling, and Visualization. DOI.
- Rieger, J., Hornig, N., Flossdorf, J., Müller, H., Mündges, S., Jentsch, C., Rahnenführer, J. and Elmer, C. (2023). Debunking Disinformation with GADMO: A Topic Modeling Analysis of a Comprehensive Corpus of German-language Fact-Checks. Proceedings of the 4th Conference on Language, Data and Knowledge. Link. GitHub.
- Rieger, J., Hornig, N., Schmidt, T. and Müller, H. (2023). Early Warning Systems? Building Time Consistent Perception Indicators for Economic Uncertainty and Inflation Using Efficient Dynamic Modeling. Proceedings of the 3rd Workshop on Modelling Uncertainty in the Financial World. Link. GitHub.
- Bittermann, A. and Rieger, J. (2022). Finding scientific topics in continuously growing text corpora. Proceedings of the 3rd Workshop on Scholarly Document Processing. Link. GitHub. PsychTopics App. Poster.
- Lange, K.-R., Rieger, J., Benner, N. and Jentsch, C. (2022). Zeitenwenden: Detecting changes in the German political discourse. Proceedings of the 2nd Workshop on Computational Linguistics for Political Text Analysis. pdf. GitHub.
- Rieger, J., Lange, K.-R., Flossdorf, J. and Jentsch, C. (2022). Dynamic change detection in topics based on rolling LDAs. Proceedings of the Text2Story'22 Workshop. CEUR-WS 3117, 5-13. pdf. GitHub.
- Rieger, J., Jentsch, C. and Rahnenführer, J. (2021). RollingLDA: An Update Algorithm of Latent Dirichlet Allocation to Construct Consistent Time Series from Textual Data. Findings of the Association for Computational Linguistics: EMNLP 2021, 2337-2347. DOI. GitHub.
- von Nordheim, G., Rieger, J. and Kleinen-von Königslöw, K. (2021). From the fringes to the core – An analysis of right-wing populists’ linking practices in seven EU parliaments and Switzerland. Digital Journalism. DOI. GitHub. EJO.
- von Nordheim, G., Koppers, L., Boczek, K., Rieger, J., Jentsch, C., Müller, H. and Rahnenführer, J. (2021). Die Entwicklung von Forschungssoftware als praktische Interdisziplinarität. M&K Medien & Kommunikationswissenschaft 69, 80-96. DOI.
- Rieger, J., Jentsch, C. and Rahnenführer, J. (2020). Assessing the Uncertainty of the Text Generating Process using Topic Models. ECML PKDD 2020 Workshops. CCIS 1323, 385-396. DOI. GitHub.
- Rieger, J. (2020). ldaPrototype: A method in R to get a Prototype of multiple Latent Dirichlet Allocations. Journal of Open Source Software 5(51), 2181. DOI.
- Rieger, J., Rahnenführer, J. and Jentsch, C. (2020). Improving Latent Dirichlet Allocation: On Reliability of the Novel Method LDAPrototype. Natural Language Processing and Information Systems, NLDB 2020. LNCS 12089, 118-125. DOI.
- von Nordheim, G. and Rieger, J. (2020). Distorted by Populism – A computational analysis of German parliamentarians’ linking practices on Twitter [Im Zerrspiegel des Populismus – Eine computergestützte Analyse der Verlinkungspraxis von Bundestagsabgeordneten auf Twitter]. Publizistik 65, 403-424. DOI. GitHub. EJO.
Recent Submissions
- Rieger, J., Ruckdeschel, M. and Wiedemann, G.: PETapter: Leveraging PET-style classification heads for modular few-shot parameter-efficient fine-tuning. OpenReview.
- Loschke C., Braungardt, S. and Rieger, J.: What motivates and demotivates energy savings in times of crisis? - An argument mining analysis using X/Twitter data. DOI.
- Schmidt, T., Rieger, J., Rahnenführer, J., Viciano, A. and Wormer, H.: Questions of quality: How AI can help to improve science communication.
Working Papers
- Schmidt, T., Müller, H., Rieger, J., Schmidt, T. and Jentsch, C. (2023). Inflation Perception and the Formation of Inflation Expectations. Ruhr Economic Papers #1025. DOI.
- Shrub, Y., Rieger, J., Müller, H. and Jentsch, C. (2022). Text data rule - don't they? A study on the (additional) information of Handelsblatt data for nowcasting German GDP in comparison to established economic indicators. Ruhr Economic Papers #964. DOI.
Non-peer-reviewed publications
- Müller, H., Schmidt, T., Rieger, J., Hornig, N. and Hufnagel, L.M. (2023). The Inflation Attention Cycle: Updating the Inflation Perception Indicator (IPI) up to February 2023 - a Research Note. DoCMA Working Paper #13. DOI. GitHub.
Previous editions and further links: Handelsblatt (07/25/2022), An Increasing Sense of Urgency (06/30/2022), Handelsblatt (05/24/2022), Pressure is high (04/30/2022), Handelsblatt (03/10/2022), A German Inflation Narrative (02/28/2022). - Müller, H., Rieger, J. and Hornig, N. (2022). Vladimir vs. the Virus - a Tale of two Shocks. An Update on our Uncertainty Perception Indicator (UPI) to April 2022 - a Research Note. DoCMA Working Paper #11. DOI. GitHub.
Previous editions: "Riders on the Storm" (Q1 2021), "We’re rolling" (Q4 2020), "For the times they are a-changin'" (Q3 2020). - Jentsch, C., Mammen, E., Müller, H., Rieger, J. and Schötz, C. (2021). Text mining methods for measuring the coherence of party manifestos for the German federal elections from 1990 to 2021. DoCMA Working Paper #8. DOI. Spiegel Online.
- Rieger, J. and von Nordheim, G. (2021). corona100d – German-language Twitter dataset of the first 100 days after Chancellor Merkel addressed the coronavirus outbreak on TV. DoCMA Working Paper #4. DOI. GitHub.
Other Publications
- Bittermann, A., Müller, S. M., and Rieger, J. (2022). PsychTopics: How to keep track of the psychology research landscape [PsychTopics: Wie man den Überblick über die Forschungslandschaft der Psychologie behält]. Open Password. Link.
- Rieger, J. (2019). Mónica Bécue-Bertaut (2019): Textual Data Science with R. Statistical Papers 60, 1797-1798. DOI.
Dissertation
- Rieger, J. (2022). Reliability evaluation and an update algorithm for the latent Dirichlet allocation. Dissertation. TU Dortmund University. DOI.
Invited talks
- Detecting narration changes in economics utilizing continuous topic modeling and (large) language models. Research Colloquium of the Faculty of Business Studies and Economics, JLU. Gießen, Germany (01/2025).
- Monitoring (social) media narratives combining retrospective few-shot classification with continuous topic modeling. CMStatistics 2024. London, England (12/2024).
- PETapter: A masked-language-modeling classification head for modular fine-tuning of (large) language models. CompStat 2024. Gießen, Germany (08/2024).
- Bekämpfung von Desinformation und Fake-News durch GADMO. BDI/BDA-Arbeitskreis Statistik. Düsseldorf, Germany (05/2024).
- Keep rollin’! The abilities for monitoring growing corpora using RollingLDA. ZPID Lecture Series. Trier, Germany (12/2022). Recording.
Contributions to conferences and workshops
- Exploring the potential of large language models (such as GPT-4) for (semi-)automatic content analysis of stances and frames in media texts. DGPuK 2024. Erfurt, Germany (03/2024). Abstract.
- Few-shot learning for automated content analysis (FLACA) in the German media debate on arms deliveries to Ukraine. Digital Total. Hamburg, Germany (10/2023). Poster.
- Debunking Disinformation with GADMO: A Topic Modeling Analysis of a Comprehensive Corpus of German-language Fact-Checks. DiTox'23 Workshop @LDK 2023. Vienna, Austria (09/2023).
- Bekämpfung von Desinformation durch GADMO: Analyse eines umfassenden deutschsprachigen Faktencheck-Korpus mithilfe von Topic Modellen. Statistische Woche 2023. Dortmund, Germany (09/2023).
- Scrutinizing ChatGPT against Few-Shot Learning with Adapter Extensions and XLM-RoBERTa: A Case Study on Identifying Claims, Arguments and their Stance in the German News Media Debate on Arms Deliveries to Ukraine. Statistische Woche 2023. Dortmund, Germany (09/2023).
- Beyond "Master Frames": A Semi-automated Approach to Studying Viewpoint Diversity of the Media Discourse. ECREA PolComm 2023. Berlin, Germany (08/2023).
- Few-shot learning for automated content analysis: Efficient coding of arguments and claims in the debate on arms deliveries to Ukraine. DGPuK 2023. Bremen, Germany (05/2023).
- Early Warning Systems? Building Time Consistent Perception Indicators for Economic Uncertainty and Inflation Using Efficient Dynamic Modeling. MUFin'23 Workshop @AAAI 2023. Washington, DC, USA (02/2023). Pre-recording.
- Finding scientific topics in contionuously growing text corpora. SDP'22 Workshop @COLING 2022. Gyeongju, Republic of Korea (10/2022). Pre-recording.
- Monitoring consistent topics in continuously growing scientific text corpora. Statistische Woche 2022. Münster, Germany (09/2022).
- Dynamic change detection in topics based on rolling LDAs. Text2Story'22 Workshop @ECIR 2022. Stavanger, Norway (04/2022). Pre-recording.
- Improving the reliability of LDA results using LDAPrototype as selection criterion. DAGStat 2022. Hamburg, Germany (03/2022).
- RollingLDA: An Update Algorithm of Latent Dirichlet Allocation to Construct Consistent Time Series from Textual Data. EMNLP 2021. Punta Cana, Dominican Republic (11/2021). Pre-recording.
- Assessing the Uncertainty of the Text Generating Process using Topic Models. EDML'20 Workshop @ECML PKDD 2020. Online (09/2020).
- Improving Latent Dirichlet Allocation: On Reliability of the Novel Method LDAPrototype. NLDB 2020. Online (06/2020).
- Quantifizierung der Stabilität der Latent Dirichlet Allocation mithilfe von Clustering auf wiederholten Durchläufen. Statistische Woche 2019. Trier, Germany (09/2019).
- Softwaretools für die Kommunikationsforschung. DGPuK 2019. Münster, Germany (05/2019).
- Measuring Stability of Replicated LDA Runs. DAGStat 2019. Munich, Germany (03/2019).
- Natural Language Processing (WiSe 2024/25, 2023/24)
- Data Mining Cup (SuSe 2024, 2023, 2022, 2021, 2020, 2019)
- Text as Data (WiSe 2022/23)
- Einführung in LaTeX (SuSe 2022, 2021, 2020, 2019)
- Fallstudien I (WiSe 2022)
- Schätzen und Testen I (WiSe 2022)
- Nichtparametrische Verfahren (WiSe 2021)
- Seminar: Text Data meets Econometrics (WiSe 2021)
- Entscheidungstheorie - Statistik VI (SuSe 2020)
- Wahrscheinlichkeitstheorie - Statistik V (WiSe 2020)
- Seminar: Textdatenanalyse (SuSe 2019)