M.Sc. Maxime Faymonville
Kontakt
Technische Universität Dortmund
Fakultät Statistik
Lehrstuhl für Wirtschafts- und Sozialstatistik
CDI-Gebäude, Raum 4
44221 Dortmund
E-Mail: faymonvillestatistik.tu-dortmundde
Tel.: +49 231 755 5203
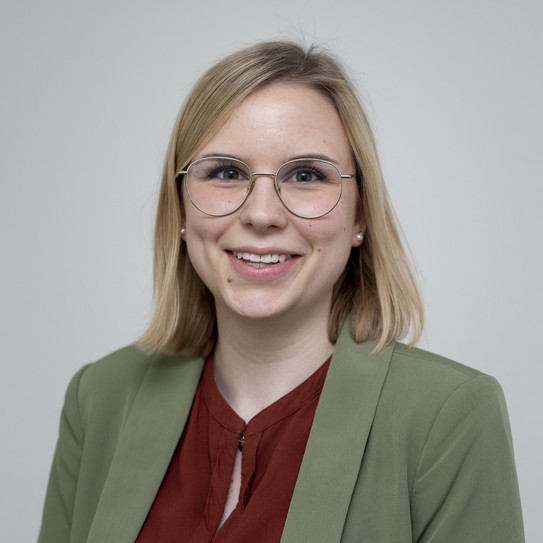
- seit Januar 2021: Wissenschaftliche Mitarbeiterin und Promovierende
- 2023: Studienkoordinatorin der Fakultät Statistik
- 2018-2020: Studentische bzw. wissenschaftliche Hilfskraft am Lehrstuhl für Wirtschafts- und Sozialstatistik
- 2018-2020: M.Sc. Statistik mit Schwerpunkt Ökonometrie, TU Dortmund
Masterarbeit: Bootstrap-based prediction for INAR processes - 2015-2018: B.Sc. Statistik mit Schwerpunkt Ökonometrie, TU Dortmund
Mitarbeit im DFG-Projekt Modelldiagnostik für Zähldatenzeitreihen (Sachbeihilfe JE932/1-1, Projektnummer 437270842)
- Zähldatenzeitreihen
- Bootstrapverfahren
- Semiparametrische Schätzung
- spINAR (github)
- Faymonville, M., Riffo, J., Rieger, J. und Jentsch, C. (2024). spINAR: An R Package for Semiparametric and Parametric Estimation and Bootstrapping of Integer-Valued Autoregressive (INAR) Models. Journal of Open Source Software, 9(97), 5386. DOI.
- Aleksandrov, B., Weiß, C.H., Nik, S., Faymonville, M. und Jentsch, C. (2023): Modelling and Diagnostic Tests for Poisson and Negative-binomial Count Time Series. Metrika. DOI.
- Weiß, C.H., Aleksandrov, B., Faymonville, M. und Jentsch, C. (2023): Partial Autocorrelation Diagnostics for Count Time Series. Entropy 2023, 25(1), 105. DOI.
- Aleksandrov, B., Weiß, C.H., Jentsch, C. und Faymonville, M. (2022). Novel Goodness-of-Fit Tests for Binomial Count Time Series. Statistics, 56(5), 957-990. DOI.
- Faymonville, M., Jentsch, C., Weiß, C.H. und Aleksandrov, B. (2022). Semiparametric Estimation of INAR Models using Roughness Penalization. Statistical Methods & Applications 32(2), 365-400. DOI.
Aktuelle Einreichungen
- Faymonville, M., Jentsch, C. und Weiß, C.H. (2024). Semi-parametric Goodness-of-fit Testing for INAR Models. arXiv.
- Joint vector-autoregressive modeling of real- and integer-valued time series with full autoregressive parameter range. IMS 2024. Bochum, Deutschland (08/2024).
- Predictive inference for count data time series. SMSA 2024. Delft, Niederlande (03/2024).
- Goodness-of-fit testing for INAR models. CFE 2023. Berlin, Deutschland (12/2023).
- Goodness-of-fit testing for INAR models. Kolloquium der Fächergruppe Mathematik und Statistik. Hamburg, Deutschland (10/2023).
- Predictive inference for count data time series. Statistische Woche 2023. Dortmund, Deutschland (09/2023).
- Joint semiparametric INAR bootstrap inference for model coefficients and innovation parameters. GPSD 2023. Essen, Deutschland (03/2023).
- Joint semiparametric INAR bootstrap inference for model coefficients and innovation parameters. CFE 2022. London, Großbritannien (12/2022).
- Joint semiparametric INAR bootstrap inference for model coefficients and innovation parameters. Statistische Woche 2022. Münster, Deutschland (09/2022).
- Semiparametric estimation of INAR models using roughness penalization. DAGStat 2022. Hamburg, Deutschland (03/2022).
- Bootstrap Methods (SoSe 2024)
- Econometrics (SoSe 2023)
- Vektor- und Matrizenrechnung (WiSe 2022/23)
- Econometrics (SoSe 2022)
- Bootstrap Methods (WiSe 2021/22)
- Econometrics (SoSe 2021)
- Financial Econometrics (WiSe 2020/21)
- Lineare Modelle (SoSe 2019)
- Analysis I Tutorium für Statistiker (WiSe 2017/18)
- Analysis II Tutorium für Statistiker (SoSe 2017)
- Analysis I Tutorium für Statistiker (WiSe 2016/17)