Prof. Dr. Carsten Jentsch
Kontakt
Technische Universität Dortmund
Fakultät Statistik
Lehrstuhl für Wirtschafts- und Sozialstatistik
CDI-Gebäude, Raum 9
44221 Dortmund
E-Mail: jentschstatistik.tu-dortmundde
Tel.: +49 231 755 3869
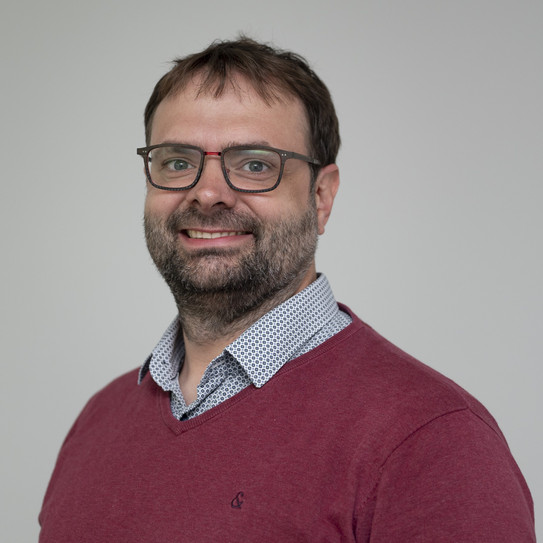
Carsten Jentsch hat 2001-2007 Mathematik mit Nebenfach BWL an der TU Braunschweig studiert, wo er 2010 auch promoviert wurde. Nach einem Forschungsaufenthalt an der UC San Diego wurde er 2011 Postdoc an der VWL-Fakultät der Universität Mannheim und am SFB 884 The Political Economy of Reforms. Seit 2015 ist er Mitglied im Eliteprogramm für Postdocs der Baden-Württemberg Stiftung. Nach Professurvertretungen an den Universitäten Bayreuth und Mannheim arbeitet er seit Sommersemester 2018 an der TU Dortmund. Er ist Mitglied der RGS Faculty an der Ruhr Graduate School in Economics. Seit 2020 ist er stellvertretender Vorsitzender des Ausschusses Empirische Wirtschaftsforschung und Angewandte Ökonometrie in der Deutschen Statistischen Gesellschaft.
Die Forschungsinteressen von Carsten Jentsch liegen im Bereich der mathematischen Statistik mit Schwerpunkt auf der methodischen Entwicklung und Implementierung von Schätz- und Testverfahren sowie auf der Modellierung von zeitlich und/oder räumlich abhängigen Daten und deren Anwendung in den Wirtschafts- und Sozialwissenschaften. Er beschäftigt sich mit verschiedenen Themen aus der Zeitreihenanalyse bzw. Zeitreihenökonometrie, wobei er verstärkt Methoden aus dem Spektralbereich verwendet. Insbesondere sind Bootstrapverfahren für abhängige Daten ein wesentlicher Gegenstand seiner Forschung. Weiterhin interessiert er sich für statistische Methoden für stochastische Netzwerke und die statistische Analyse von Textdaten.
- Editor-In-Chief für Statistical Papers (seit 2018)
- Associate Editor für Journal of Time Series Analysis (seit 2019)
- Associate Editor für Statistics (2018-2020)
- Associate Editor für Statistics & Risk Modeling (2017-2020)
- Associate Editor für Statistics & Probability Letters (2016-2020)
- Lange, K.-R., Benner, N., Grönberg, L., Hachcham, A., Kolli, I., Rieger, J. und Jentsch, C. (2025). ttta: Tools for Temporal Text Analysis. arXiv. Link.
- Dzikowski, D. und Jentsch, C. (2024). Structural Periodic Vector Autoregressions. arXiv.
- Schmidt, T., Müller, H., Rieger, J., Schmidt, T. und Jentsch, C. (2024). Changing inflation perception and the propagation of news shocks.
- Krabel, M. T., Tien Tran, T. N., Groll, A., Horn, D. und Jentsch, C. (2022). Random Depth Boosting and Random Depth Forests - A Random Tree Depth Injection Approach. Alte Version
- Shrub, Y., Rieger, J., Müller, H. und Jentsch, C. (2022). Text data rule - don't they? A study on the (additional) information of Handelsblatt data for nowcasting German GDP in comparison to established economic indicators. Ruhr Economic Papers #964. DOI. Link.
- Lange, K.-R., Reccius, M., Schmidt, T., Müller, H., Roos, M., und Jentsch, C. (2022). Towards extracting collective economic narratives from texts. Ruhr Economic Papers #963. Link
- Benner, N., Lange, K.-R., und Jentsch, C. (2022). Named Entity Narratives. Ruhr Economic Papers #962. Link.
- Flossdorf, J., Meyer, A., Artjuch, D., Schneider, J. und Jentsch, C.: Unsupervised Movement Detection in Indoor Positioning Systems. arXiv.
2025
- Lange, K.-R., Schmidt, T., Reccius, M., Müller, H., Roos, M. und Jentsch, C. (2025). Narrative Shift detection: A hybrid approach of Dynamic Topic Models and Large Language Models. Erscheint in Proceedings of the Text2Story'25 Workshop.
- Müller, H., Blagov, B., Schmidt, T., Rieger, J. und Jentsch, C. (2025). The macroeconomic impact of asymmetric uncertainty shocks. The Journal of Economic Asymmetries , 31(e00410). DOI.
2024
- Faymonville, M., Jentsch, C. und Weiß, C.H. (2024). Semi-parametric Goodness-of-fit Testing for INAR Models. Akzeptiert für Bernoulli.
- Lange, K.-R., Rieger, J. und Jentsch, C. (2024). Lex2Sent: A bagging approach to unsupervised sentiment analysis. Proceedings of the 20th Conference on Natural Language Processing (KONVENS 2024), 281-291. pdf. arXiv. GitHub.
- Brune, B., Flossdorf, J. und Jentsch, C. (2024). Goodness-of-fit Testing based on Graph Functionals for Homogeneous Erdös-Rényi Graphs. Scandinavian Journal of Statistics, 1-49. arXiv.
- Rieger, J., Jentsch, C. und Rahnenführer, J. (2024): LDAprototype: A Model Selection Algorithm to Improve Reliability of Latent Dirichlet Allocation. PeerJ Computer Science, 10(2279).
- Faymonville, M., Riffo, J., Rieger, J. und Jentsch, C. (2024). spINAR: An R Package for Semiparametric and Parametric Estimation and Bootstrapping of Integer-Valued Autoregressive (INAR) Models. Journal of Open Source Software, 9(97), 5386. DOI.
- Dorn, M., Birke, M. und Jentsch, C. (2024). Testing exogeneity in the functional linear regression model. Akzeptiert für Statistica Sinica. arXiv.
- Steinmetz, J. und Jentsch, C. (2024). Bootstrap Consistency for the Mack Bootstrap. Insurance: Mathematics and Economics, 115, 83-121. DOI.
- Aleksandrov, B., Weiß, C.H., Nik, S., Faymonville, M. und Jentsch, C. (2024). Modelling and Diagnostic Tests for Poisson and Negative-binomial Count Time Series. Metrika, 87, 843-887. DOI.
2023
- Reichold, K. und Jentsch, C. (2023). Bootstrap Inference in Cointegrating Regressions: Traditional and Self-Normalized Test Statistics. Journal of Business & Economic Statistics, 47(3), 970-983. arXiv. DOI.
- Lange, K.-R., Jentsch, C. (2023). SpeakGer: A meta-data enriched speech corpus of German state and federal parliaments. Proceedings of the 3rd Workshop on Computational Linguistics for Political Text Analysis, 19–28. pdf. download.
- Krause, C., Rieger, J., Flossdorf, J., Jentsch, C. und Beck, F. (2023). Visually Analyzing Topic Change Points in Temporal Text Collections. Proceedings of the Conference on Vision, Modeling, and Visualization. DOI.
- Rieger, J., Hornig, N., Flossdorf, J., Müller, H., Mündges, S., Jentsch, C., Rahnenführer, J. und Elmer, C. (2023). Debunking Disinformation with GADMO: A Topic Modeling Analysis of a Comprehensive Corpus of German-language Fact-Checks. Proceedings of the 4th Conference on Language, Data and Knowledge, 520–531. pdf. GitHub.
- Flossdorf, J., Fried, R. und Jentsch, C. (2023). Online Monitoring of Dynamic Networks using flexible Multivariate Control Charts. Social Network and Analysis Mining, 13, 87. DOI.
- Walsh, C. und Jentsch, C. (2023). Nearest Neighbor Matching: M-out-of-N Bootstrapping without Bias Corrections vs. the Naive Bootstrap. Erscheint in Econometrics and Statistics. DOI.
- Weiß, C.H., Aleksandrov, B., Faymonville, M. und Jentsch, C. (2023). Partial Autocorrelation Diagnostics for Count Time Series. Entropy 2023, 25(1), 105. DOI.
2022
- Jentsch, C. und Lunsford, K. (2022). Asymptotically valid Bootstrap Inference for Proxy SVARs. Journal of Business & Economic Statistics 40, 4, 1876-1891. DOI. Supplement. Code.
- Li, B., Jentsch, C. und Müller, E. (2022). Prototypes as Explanation for Time Series Anomaly Detection. Proceedings of the ANDEA 2022 Workshop. pdf.
- Aleksandrov, B., Weiß, C.H., Jentsch, C. und Faymonville, M. (2022). Novel Goodness-of-Fit Tests for Binomial Count Time Series. Statistics, 56(5), 957-990. DOI.
- Steinmetz, J. und Jentsch, C. (2022). Asymptotic Theory for Mack's Model. Insurance: Mathematics and Economics, 107, 223-268. DOI.
- Faymonville, M., Jentsch, C., Weiß, C.H. und Aleksandrov, B. (2022). Semiparametric Estimation of INAR Models using Roughness Penalization. Statistical Methods & Applications 32(2), 365-400. DOI.
- Lange, K.-R., Rieger, J., Benner, N. und Jentsch, C. (2022). Zeitenwenden: Detecting changes in the German political discourse. Proceedings of the 2nd Workshop on Computational Linguistics for Political Text Analysis, 47-53. pdf. GitHub.
- Rieger, J., Lange, K.-R., Flossdorf, J. und Jentsch, C. (2022). Dynamic change detection in topics based on rolling LDAs. Proceedings of the Text2Story'22 Workshop. CEUR-WS 3117, 5-13. pdf. GitHub.
- Jentsch, C. und Reichmann, L. (2022). Generalized Binary VAR Processes. Journal of Time series Analysis 43, 285-311. DOI.
2021
- Rieger, J., Jentsch, C. und Rahnenführer, J. (2021). RollingLDA: An Update Algorithm of Latent Dirichlet Allocation to Construct Consistent Time Series from Textual Data. Findings of the Association for Computational Linguistics: EMNLP 2021, 2337-2347. DOI. GitHub.
- Flossdorf, J. und Jentsch, C. (2021). Change Detection in Dynamic Networks Using Network Characteristics. IEEE Transactions on Signal and Information Processing over Networks 7, 451-464. DOI.
- Aleksandrov, B., Weiß, C.H. und Jentsch, C. (2021): Goodness-of-fit Tests for Poisson Count Time Series based on the Stein-Chen Identity. Statistica Neerlandica 76(1), 35-64. DOI.
- Jentsch, C., Lee, E. R. und Mammen, E. (2021). Poisson reduced rank models with an application to political text data. Biometrika 108(2), 455-468. DOI.
2020
- Jentsch, C. und Kulik, R. (2020). Bootstrapping Hill estimator and tail array sums for regularly varying time series. Bernoulli 27(2), 1409-1439. DOI.
- Rieger, J., Jentsch, C. und Rahnenführer, J. (2020). Assessing the Uncertainty of the Text Generating Process using Topic Models. ECML PKDD 2020 Workshops. CCIS 1323, 385-396. DOI. GitHub.
- Jentsch, C. und Meyer, M. (2020). On the validity of Akaike's identity for random fields. Journal of Econometrics 222(1C), 676-687. DOI.
- Rieger, J., Rahnenführer, J. und Jentsch, C. (2020). Improving Latent Dirichlet Allocation: On Reliability of the Novel Method LDAPrototype. Natural Language Processing and Information Systems, NLDB 2020. LNCS 12089, 118-125. DOI.
- Jentsch, C., Lee, E. R. und Mammen, E. (2020). Time-dependent Poisson reduced rank models for political text data analysis. Computational Statistics and Data Analysis 142, 106813. DOI.
- Jentsch, C., Leucht, A., Meyer, M., und C. Beering (2020). Empirical characteristic functions-based estimation and distance correlation for locally stationary processes. Journal of Time Series Analysis 41, 110-133. DOI.
2019
- Jentsch, C. und Reichmann, L. (2019). Generalized Binary Time Series Models. Econometrics 7, 47. DOI.
- Jentsch, C. und Lunsford, K. (2019). The Dynamic Effects of Personal and Corporate Income Tax Changes in the United States: Comment. American Economic Review 109(7), 2655-2678. DOI. Supplement. Code.
- Weiß, C. H. und Jentsch, C. (2019). Bootstrap-based Bias Corrections for INAR Count Time Series. Journal of Statistical Computation and Simulation 89(7), 1248-1264. DOI.
- Jentsch, C. und Weiß (2019), C. H.. Bootstrapping INAR models. Bernoulli 25(3), 2359-2408. DOI. Working Paper.
2018
- Weiß, C. H., Steuer, D., Jentsch, C. und Testik, M. C. (2018). Guaranteed Conditional ARL Performance in the Presence of Autocorrelation. Computational Statistics and Data Analysis 128, 367-379. DOI.
2017
- Meyer, M., Jentsch, C. und Kreiss, J.-P. (2017). Baxter's Inequality and Sieve Bootstrap for Random Fields. Bernoulli 23(4B), 2988-3020. DOI. Working Paper.
- Bandyopadhyay, S., Jentsch, C. und Subba Rao, S. (2017). A spectral domain test for stationarity of spatio-temporal data. Journal of Time Series Analysis 38(2), 326-351. DOI.
2016
- Jentsch, C. und Kirch, C. (2016). How much information does dependence between wavelet coefficients contain? Journal of the American Statistical Association 111(515), 1330-1345. DOI. pdf. Code.
- Jentsch, C. und Steinmetz, J. (2016). A Connectedness Analysis of German Financial Institutions during the Financial Crisis in 2008. Banks and Bank Systems 11(4). DOI.
- Jentsch, C. und Leucht, A. (2016). Bootstrapping sample quantiles of discrete data. Annals of the Institute of Statistical Mathematics 68(3), 491-539. DOI. Working Paper.
- Brüggemann, R., Jentsch, C., und Trenkler, C. (2016). Inference in VARs with Conditional Heteroskedasticity of Unknown Form. Journal of Econometrics 191, 69-85. DOI. pdf. Working Paper.
2015
- Jentsch, C. und Politis, D. N. (2015). Covariance matrix estimation and linear process bootstrap for multivariate time series of possibly increasing dimension. The Annals of Statistics 43(3), 1117-1140. DOI. pdf. Supplement. Code.
- Jentsch, C., Paparoditis, E., und Politis, D. N. (2015). Block bootstrap theory for multivariate integrated and cointegrated time series. Journal of Time Series Analysis 36(3), 416-441. DOI. pdf.
- Jentsch, C. und Pauly, M. (2015). Testing equality of spectral densities using randomization techniques. Bernoulli 21(2), 697-739. DOI. pdf. Supplement.
- Jentsch, C. und Subba Rao, S. (2015). A test for second order stationarity of a multivariate time series. Journal of Econometrics 185(1), 124-161. DOI. pdf. Code.
2013
- Jentsch, C. und Politis, D. N. (2013). Valid resampling of higher order statistics using linear process bootstrap and autoregressive sieve bootstrap. Communications in Statistics - Theory and Methods 42(7), 1277-1293. pdf.
2012
- Jentsch, C., Kreiss, J.-P., Mantalos, P. und Paparoditis, E. (2012). Hybrid bootstrap aided unit root testing. Computational Statistics 27(4), 779-797. DOI.
- Jentsch, C. (2012). A new frequency domain approach of testing for covariance stationarity and for periodic stationarity in multivariate linear processes. Journal of Time Series Analysis 33(2), 177-192. DOI. pdf.
- Jentsch, C. und Mammen, E. (2012). Discussion on the paper "Bootstrap for dependent data: A review" by Jens-Peter Kreiss and Efstathios Paparoditis. Journal of the Korean Statistical Society 40(4), 391-392. DOI.
- Jentsch, C. und Pauly, M. (2012). A note on periodogram-based distances for comparing spectral densities. Statistics and Probability Letters 82(1), 158-164. DOI. pdf.
2010
Discussion Papers
- Blagov, B., Müller, H., Jentsch, C. und Schmidt, T.: The Investment Narrative - Improving Private Investment Forecasts with Media data. Ruhr Economic Papers #921. Link.
- Jentsch, C., Müller, H., Mammen, E., Rieger, J. und Schötz, C.: Text mining methods for measuring the coherence of party manifestos for the German federal elections from 1990 to 2021. DoCMA Working Paper #8. DOI.
- Krabel, T. M., Tran, T.N.T., Groll, A., Horn, D. und Jentsch, C.: Random boosting and random^2 forest – A random tree depth injection approach. arXiv.
- Walsh, C., Jentsch, C. und Hossain, S.T.: Nearest neighbor matching: Does the M-out-of-N bootstrap work when the naïve bootstrap fails? SFB 823 Discussion Paper 2021(5). DOI.
- Walsh, C., Jentsch, C. und Hossain, S.T.: Weighted bootstrap consistency for matching estimators: the role of bias-correction. SFB 823 Discussion Paper 2021(8). DOI.
Sonstige Veröffentlichungen
- Groll, A. und Jentsch, C. (2022). Über "Paola Zuccolotto and Marica Manisera (2020): Basketball Data science: With Applications in R." Statistical Papers 63, 991-993. DOI.
- Jentsch, C., Müller, H., Mammen, E., Rieger, J. und Schötz, C. (2021, 18. September). Textanalyse ergibt mögliche Koalitionen: Wer zusammen passt – und wer nicht. Spiegel Online. Institut für Journalistik.
- von Nordheim, G., Koppers, L., Boczek, K., Rieger, J., Jentsch, C., Müller, H. und Rahnenführer, J. (2021). Die Entwicklung von Forschungssoftware als praktische Interdisziplinarität. M&K Medien & Kommunikationswissenschaft 69, 80-96. DOI.
- Rahnenführer, J. und Jentsch, C. (2019). Wer soll das alles lesen? Automatische Analyse von Textdaten. In: Faszination Statistik. Einblicke in aktuelle Forschungsfragen und Erkenntnisse. Eds. Krämer W. und Weihs, C., 191-199. DOI.
- Jentsch, C. und Politis, D.N. (2011). The multivariate linear process bootstrap. Proceedings of the 17th European Young Statisticians Meeting (EYSM). pdf.
- Jentsch, C. (2010). (Hybride) Bootstrapverfahren - Wie konstruiert man gute Konfidenzintervalle? In: Theorie und Anwendung lernender Algorithmen in den Ingenieurs- und Naturwissenschaften an der TU Braunschweig. Eds. Heinert, M. und Riedel, B., Geod. Schriftr. TU Braunschweig 25; 27-32.